The Health and Safety profession has made great strides in safety performance over the last 50 years, technology has become safer to use, and techniques such as HAZOP and bowtie analysis have given us ways to think about and manage hazards. This approach has served the industry well, but safety performance has recently stalled.
What more can we do to achieve the next step change in safety performance?
One problem is that when we define safety as a lack of incidents, a lack of incidents also means a lack of data. Incidents can appear random, with ten serious incidents this year, three last year and who knows how many next year. Even if we have perfect procedures, perfect equipment and ideal training, the workplace is constantly changing.
Workers' reality can be very different from what was initially planned. This leads to a difference between work as imagined and work as done, or in other words, variation in performance.
To illustrate this, imagine a lifting job. The task is to lift an i-beam to a structure and fit it into a slot. Sometimes the space is big enough, sometimes too small, and workers find that they may have to force the beam in by pushing it. This could make the beam swing uncontrollably, and potentially it could even fall from the slings. For nine of the i-beams, this approach could work without incident, but on the tenth occasion, the i-beam falls. We would have nine good outcomes and one bad outcome, yet the same variation in performance. The difference between work as imagined and work as done was present regardless of the outcome.
Instead of measuring the outcome, modern approaches to safety are increasingly focusing on measuring and learning from these variations in normal work directly. The UK rail industry has taken this approach to address signals passed at danger (SPAD). When the next section of track is not clear, a train driver must stop before passing a red signal or risk colliding with another train further up the line. When a train passes a red signal, this is called a SPAD.
A train operator wanted to know if there were clear differences between drivers that passed red signals and those that didn't. To do this, they decided to measure the stopping distance of each train after encountering a red signal. They expected a clear difference in stopping distances between when things went right and when SPADS occurred, but they didn't.
There was no apparent difference in the behaviour of drivers. Instead, they saw a wide variation in stopping distances and a normal distribution curve. The stopping distances were occasionally greater than is allowable, and a SPAD occurred. It was concluded that rather than being exceptional events, SPADS were simply a result of having such a wide variation in braking distances and therefore were expected to occur under the current operating conditions.
Several factors caused the wide variation in braking distances.
- Drivers were encouraged not to slow down unnecessarily to save on electricity and fuel costs.
- Drivers would delay braking as long as possible because they expected the signal to change to green, but they had no way of knowing this.
By providing trained drivers with information about the status of signals further up the line, drivers were better able to predict whether a signal would change to green or not and so adjusted their behaviour accordingly. The result was that breaking distances became more uniform, resulting in fewer SPADS.
So what can we learn from this? There will always be a difference between work as imagined and work as done, and there will always be performance variability. This is normal and even vital for organizations to be flexible and adaptable.
When incidents happen, these may be exceptional outcomes but not unusual ones.
It is unhelpful to blame someone or put these down to bad luck. While our systems can cope with variation up to a point, the difference between a safe outcome and a dangerous one is closer than we think. By ignoring normal work, we miss 99% of the data. Let's move away from thinking about safety as a binary outcome and instead find ways to measure variation in performance before incidents happen directly. We can predict where incidents are likely to occur. These will be the areas of work where our people and processes experience wide variations in performance beyond which our systems can cope. Whether our process is driving trains or creating fuels and generating energy, this is true.
Begin by looking at your performance indicators for safety-critical activities. Are they only measuring the outcome? What do they tell you about the variation in performance that leads to that outcome? For example, instead of measuring whether routine maintenance is completed to schedule, you could try to count how many days before or after the scheduled maintenance is completed. What might this tell you about how organised or well-resourced the maintenance program is? Instead of measuring how often operating temperature levels exceed a certain threshold, you could try to measure the variation in temperature relative to that threshold. How close to the edge are you working?
Lastly, suppose leaders recognise and start discussing the variation between work as imagined and work as done. In that case, this may help workers recognize this variation before the job starts, and better prepare them to stop and replan.
Organisational Resilience is achievable with thoughtful analysis mediated by operational expertise and adequate resourcing.
(Adapted from an article originally written by the UK Energy Institute)
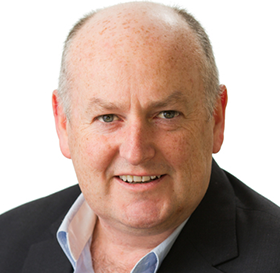